Become a Large Language Model! Teaching Text Generation with an Analog Game
,
Colorado Convention Center, Bluebird Ballroom Lobby, Table 28
Presenters
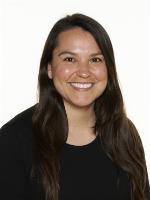
Session description
Purpose & objective
Participants will play a quick game, where they "become a large language model," to enhance their understanding of tokenization in generative AI.
Participants will also view images and descriptions of how this strategy has been used in teacher professional development sessions. Coaches will come away with the skills to replicate this strategy with their staff.
Teacher participants will come away with a strategy they can use with students.
The aim of this is to address the misunderstandings of educators and students in their use of large language models. Many teachers I've worked with have tried a large language model and come away frustrated, or confused about what can be done. Teachers have told me their frustrations when they've asked it to write music, or in iambic pentameter, or problems with specific math functions. I've come to realize that often, these misunderstandings could be challenged with more information about how large language models work. Once we understand more about how they work, we understand a few things better- why some of our prompts aren't effective, why AI detectors aren't currently trustworthy, and how good generative AI actually is at what it is made to do! When we understand how it works, and works well, we might be able to better see how big the impact on the world will be. And, in my view, we need teachers to understand the influence that generative AI will have on the world, because we need to prepare our students for it!
I struggled to find resources to teach educators the way I needed to- in a quick, low-barrier, engaging way, so I designed this activity to support my faculty's learning. The feedback has been that teachers came away with a greater understanding of AI, it prompted conversations at home, and encouraged their exploration of the topic on their own.
In the poster presentation, I hope to have images and descriptions of how the demonstrated strategy has been used in teacher training, materials that will support the lessons, along with the opportunity to gather 3-5 passers by to play the quick game.Participants will "become" a large language model and work collaboratively to emulate the tokenization and generative process of an LLM. No devices are needed to play.
The poster presentation will succeed if several people walk away with a tool which they plan to use to engage teachers in conversations or in class with students.
Outline
Content: The two main elements will be a poster description of how I have used the "Become a Large Language Model" strategy in teacher professional development session, along with the opportunity to engage in the game, to demonstrate how this looks when teaching this in groups.
Time: Viewers could take no longer than 5 minutes to engage with the images. The interactive game portion will also take about 5 minutes for those who choose to participate.
Process: Viewers who approach the booth will have the opportunity to view the poster and ask questions. When I am able to gather several participants, we can play the game. I hope to be able to model the game every 10 minutes during the 90 minute poster presentation session.
Supporting research
https://www.researchgate.net/publication/369385210_Education_in_the_Era_of_Generative_Artificial_Intelligence_AI_Understanding_the_Potential_Benefits_of_ChatGPT_in_Promoting_Teaching_and_Learning
This article shows that there is a great demand in understanding how AI will be used in teaching and learning. We know there is also the barrier of confidence for many educators in learning about this topic.
Session specifications
Change Agent
- Facilitate equitable use of digital learning tools and content that meet the needs of each learner.
- Build the capacity of educators, leaders and instructional teams to put the ISTE Standards into practice by facilitating active learning and providing meaningful feedback.
Computational Thinker
- Students understand how automation works and use algorithmic thinking to develop a sequence of steps to create and test automated solutions.