AI as a Tool for Success: Helping Preservice Teachers Benefit from AI
,
Colorado Convention Center, Bluebird Ballroom Lobby, Table 11
Presenters
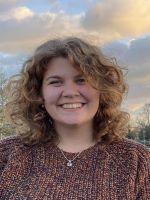
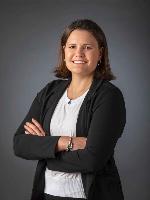
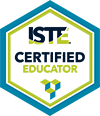
Session description
Framework
The proposed research and presentation on preparing student teachers to use AI in their classrooms is approached from multiple perspectives and theoretical frameworks, such as:
1. Constructivism: The research can be framed within a constructivist perspective, emphasizing that students actively construct their own understanding of concepts. AI are tools that help facilitate this construction by providing personalized support and resources.
2. Connectivism: This framework focuses on the idea that learning is distributed across networks of people and technology. AI is viewed as a node in this network, aiding students and teachers in accessing and synthesizing information from various sources.
3. Universal Design for Learning (UDL): UDL principles serve as a theoretical framework, emphasizing the importance of designing educational materials and environments that are accessible to all students, including those with diverse needs. AI is a tool that supports UDL by providing personalized learning pathways.
4. Social Learning Theory: This perspective considers the social aspects of learning. The research explores how AI can facilitate peer collaboration, discussions, and knowledge sharing, enhancing the social dimension of learning.
5. Ethical AI Frameworks: Ethical frameworks for AI, such as fairness, transparency, and accountability, are integrated into the research to ensure responsible AI usage in education. This perspective emphasizes the importance of ethical considerations when implementing AI technologies.
6. Innovation Adoption Theories: The research can draw from innovation adoption theories, such as the Technology Acceptance Model (TAM) or the Diffusion of Innovations theory, to understand how student teachers and educators perceive and adopt AI technologies like ChatGPT.
Methods
Data Sources:
1. Participants: Student teachers and their students in various elementary and secondary classrooms
2. Observations: Classroom observations of lessons where AI is integrated.
3. Surveys: Pre- and post-implementation surveys for student teachers to gauge their knowledge, attitudes, and self-efficacy regarding AI.
4. Interviews: Semi-structured interviews with student teachers to understand their experiences and challenges using AI.
5. Student Work: Collection of student assignments, projects, and assessments completed with AI's assistance.
Methods of Analysis:
1. Participant Selection: Student teachers will be selected from a diverse range of institutions, grade levels, and subject areas, ensuring representation.
2. Pre-Implementation Assessment: Student teachers will take a pre-implementation survey to assess their baseline knowledge and attitudes toward AI.
3. Training: Student teachers will undergo a training program covering AI's capabilities, ethical considerations, and pedagogical integration.
4. Classroom Implementation: Student teachers will integrate AI into their lessons throughout their clinical placement and/or fieldwork, with observations and student work collection.
5. Post-Implementation Assessment: After the implementation period, student teachers will complete a post-implementation survey to assess changes in knowledge and attitudes.
6. Interviews: Qualitative data from interviews will be transcribed and analyzed thematically to identify patterns and challenges faced by student teachers.
7. Student Work Analysis: Student assignments and projects will be analyzed for quality, originality, and the extent of AI's impact on outcomes.
8. Data Integration: The quantitative data from surveys and qualitative data from interviews will be integrated to provide a comprehensive understanding of the study.
9. Ethical Considerations: Ethical considerations and challenges related to AI usage will be examined in-depth.
Results
As of the proposal submission, the research study is well-conceived and actively underway. The pre-implementation phase, including participant selection, training, and initial classroom integration will be completed in January 2024. Data collection, including observations, surveys, and interviews, will be ongoing throughout the Spring 2024 semester.
While we have some preliminary insights based on initial observations and surveys, the comprehensive analysis of results, including statistical analysis of quantitative data and in-depth qualitative analysis of interviews, is yet to be completed.
The research is expected to be fully implemented and completed according to the outlined timeline.
Importance
The proposed study on preparing student teachers to use AI in their classrooms holds significant educational and scientific importance.
Educational Importance:
1. Enhancing Student Learning: The study explores the impact of AI integration on student learning outcomes. Understanding how AI technologies can positively influence student performance is crucial in the modern educational landscape.
2. Fostering Innovation: By showcasing innovative pedagogical strategies, the study encourages educators to think creatively about technology integration. It promotes a growth mindset, pushing the boundaries of traditional teaching methods.
3. Ethical Considerations: With an in-depth examination of ethical AI usage, the study contributes to ethical discussions in education. It raises awareness about responsible technology integration and data privacy, ensuring educators make informed choices.
4. Inclusivity: The study addresses the diverse needs of learners, emphasizing Universal Design for Learning principles. It provides insights into how AI can be customized to accommodate different learning styles and abilities, fostering inclusivity.
5. Teacher Preparation: Preparing student teachers to effectively use AI tools aligns with the broader goal of equipping educators with 21st-century skills. It ensures that future educators are tech-savvy and ready to meet the demands of modern classrooms.
Scientific Importance:
1. Empirical Evidence: The study contributes empirical evidence to the relatively new field of AI in education. It helps build a foundation of research-based knowledge about the benefits and challenges of AI integration in K-12 settings.
2. Validated Best Practices: By analyzing data from diverse classrooms, the study has the potential to identify best practices for integrating AI into different subject areas and grade levels, offering valuable guidance to educators.
3. Generalizability: The study's mixed-methods approach and diverse participant pool enhance the generalizability of the findings. The insights gained can inform educational practices beyond the study's specific context.
Value to ISTE Attendees:
1. Practical Insights: Attendees at ISTE 2024 will gain practical, evidence-based insights into using AI technology like AI effectively in their classrooms, aligning with ISTE's focus on technology integration.
2. Professional Development: Educators, including student teachers, will benefit from professional development opportunities presented in the study, helping them stay current in their field.
3. Ethical Considerations: Attendees will gain a deeper understanding of the ethical implications of AI in education, empowering them to make responsible technology choices.
4. Innovation Inspiration: The study encourages innovative thinking and provides concrete examples of how to harness AI for educational purposes, inspiring attendees to explore creative teaching approaches.
References
Blanka, K., Marcel, P., & Jaroslav, K. (2023). Ethical issues of the use of ai-driven mobile apps for education, 10. https://doi.org/10.3389/fpubh.2022.1118116
Brodie, K., Joffe, A., Dukhan, S., Godsell, S., de Klerk, D., & Padayachee, K. (2022). From pandemic disruption to post-pandemic transformation: new possibilities for teaching in south african higher education. South African Journal of Higher Education, 36(4), 66–84. https://doi.org/10.20853/36-4-5180
Chang, Y., Lee, S., Wong, S. F., & Jeong, S.-phil. (2022). Ai-powered learning application use and gratification: an integrative model. Information Technology & People, 35(7), 2115–2139. https://doi.org/10.1108/ITP-09-2020-0632
Essel, H. B., Vlachopoulos, D., Tachie-Menson, A., Johnson, E. E., & Baah, P. K. (2022). The impact of a virtual teaching assistant (chatbot) on students' learning in ghanaian higher education. International Journal of Educational Technology in Higher Education, 19(1). https://doi.org/10.1186/s41239-022-00362-6
Murat, E. D., Tulay, G. D., & Aras, B. (2023). The use of artificial intelligence (ai) in online learning and distance education processes: a systematic review of empirical studies, 13(3056), 3056–3056. https://doi.org/10.3390/app13053056
Inmaculada, G.-M., José, M. F.-B., Jose, F.-C., & Samuel, P. L. (2023). Analysing the impact of artificial intelligence and computational sciences on student performance: systematic review and meta-analysis, 12(1), 171–197. https://doi.org/10.7821/naer.2023.1.1240
Klimova, B., Pikhart, M., & Kacetl, J. (2023). Ethical issues of the use of ai-driven mobile apps for education. Frontiers in Public Health, 10. https://doi.org/10.3389/fpubh.2022.1118116
Reiss, M. J. (2021). The use of ai in education: practicalities and ethical considerations. London Review of Education, 19(1). https://doi.org/10.14324/LRE.19.1.05
Sdenka, Z. S.-P., Kejiang, X., & Jun, O. (2022). Artificial intelligence and new technologies in inclusive education for minority students: a systematic review, 14(13572), 13572–13572. https://doi.org/10.3390/su142013572
Zafari, M., Bazargani, J. S., Sadeghi-Niaraki, A., & Choi, S.-M. (2022). Artificial intelligence applications in k-12 education: a systematic literature review. Ieee Access, 10. https://doi.org/10.1109/ACCESS.2022.3179356
Session specifications
Laptop: PC, Chromebook, Mac
Tablet: Android, iOS, Windows
Collaborator
- Collaborate and co-learn with students to discover and use new digital resources and diagnose and troubleshoot technology issues.
Empowered Learner
- Students understand the fundamental concepts of technology operations, demonstrate the ability to choose, use and troubleshoot current technologies and are able to transfer their knowledge to explore emerging technologies.
- Students select and use digital tools to plan and manage a design process that considers design constraints and calculated risks.
Related exhibitors: | Brisk Teaching |