Unpacking the AI Mirror: Illuminating Biases in Chatbots' Stories of Learning
,
Colorado Convention Center, 108/10/12
Research papers are a pairing of two 18 minute presentations followed by 18 minutes of Discussion led by a Discussant, with remaining time for Q & A.
This is presentation 2 of 2, scroll down to see more details.
Other presentations in this group:
Presenters
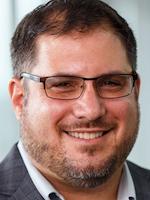
Session description
Framework
This research is informed by critical perspectives that recognize the "racecraft" inherent in the social and material fabric of our society, where, as if by magic, bias, oppression, and inequality persistently operate in our structures, relationships, actions, and dispositions unless interrogated in full light (Fields & Fields, 2014). This research calls attention to the normativity and privilege of specific identities—white, gender normative, middle or upper middle class—that allow these identities to appear as if this is the way things are supposed to be, while all other identities and communities are different (Foste & Jones, 2020; Frankenberg, 1997; Reddy, 1998). The maneuverings of AI in particular are susceptible to racecraft and normativity (Cave & Dihal, 2020), and as an emerging tool in educational spaces that are increasingly diverse (NCES, 2019), it is of critical importance to understand and interrogate the relationships between learning, teaching, and AI technologies.
Methods
The data was collected in two phases. For the first phase, data was collected via an online survey utilizing Qualtrics from faculty, staff, and students in a School of Education at a large public urban university in the American Midwest. This population was selected because all aspects of the School are driven by an antiracist and anti-oppression mission, so the discussion of factors such as race, class, and gender are neither uncommon nor uncomfortable.
The three major AI LLM chatbots in the US (Google's Bard, OpenAI's ChatGPT, and Anthropic's Claude) were provided with the following prompt:
Can you tell me about people meeting and introducing themselves to each other and then learning? They should have names and please be specific about how they each learn.
Each of the chatbots' responses to this prompt was embedded within an online survey. All responses included two people, so the participants were asked to imagine these people and identify them based on a range of identity and demographic markers, including race/ethnicity utilizing the US Census Bureau's racial and ethnic categories (American Indian and Alaska Native, Asian, Black, Latine, Native Hawaiian and Pacific Islander, or white; Jensen et al., 2021), pronouns (he/him, she/her, they/them, or other), locale utilizing the National Center for Educational Statistics (NCES) urban-centered locale categories (urban, suburban, town, or rural; NCES, 2006), and socioeconomic status utilizing the MacArthur Scale of Subjective Social Status (Adler et al., 2000; SPARQ, n.d.).
Responses from the survey were analyzed utilizing the R statistical programming language (R Core Team, 2022). Each of the characteristics were ranked through a non-parametric maximum likelihood calculation (Einbeck et al., 2018) and then assigned an a-posterior probability of appearing at that rank based on the Bayes rule (Meyer et al., 2023). This process established multi-level probabilities of the range of identity and demographic markers that each of the "characters" represented in the stories the chatbots stitched together.
The second phase involved asking the ChatGPT chatbot—the first AI LLM to be released to the general public that received sustained and wide-ranging use (Marr, 2023)—the following prompt:
Can you talk about people who {are | live in} {X} meeting each other and learning together? They should have names.
{X} represents the full range of identity and demographic markers outlined in phase 1 of the research. The stories ChatGPT stitched together were then coded at the paragraph level in two rounds, first through a frame analysis (David et al., 2011; Goffman, 1974) to identify the narrative purpose of each paragraph and then through a constant comparative coding process (Glaser, 1965) aimed at uncovering normative structures (Viruru & Rios, 2021).
Results
As I am unable to provide the tables and charts generated through the analysis, I will provide a brief overview of the findings for each phase. For phase 1 of the research, it was found that each of the characters across all three chatbots were largely coded racially as white, with Black being the second most likely assigned race/ethnicity; other identities were possibilities, but not as likely. In addition, all three chatbots stitched together a story of two people. Apart from ChatGPT, participants coded one character as preferring he/him pronouns and the other character as preferring she/her pronouns. ChatGPT's characters—"Alex" and "Bailey," where context clues beyond the names themselves influenced participants' rankings—were coded as most likely preferring they/them pronouns. All the characters were coded as upper middle class, falling on the 7th rung of the SES ladder (out of 10 rungs) with the exception of Bard's "Ben," who was identified as a software engineer originally from New York and placed on rung 8, and Claude's "David," who the chatbot identified as a high school math teacher from Detroit and was placed on rung 6 by participants.
For phase 2, it was found that ChatGPT's stories of learning followed a predictable narrative frame pattern: 1) Introduction, 2) Encounter, 3) Public Program Attendance, 4) Cultural/Civic Participation, 5) Books and Media, 6) Extension of Learning, 7) Translation into Action, and 8) Conclusion. Exceptions did exist. For example, white learners engaged in networking while other racial/ethnic identities did not. From a content perspective, almost all groups engaged in some sort of emancipatory or political activism during the Translation into Action stage with the exception of men (who took up hobbies) and people with advanced degrees (upper SES, who mentored students). The two lower SES characters were named "Maria" and "Carlos," both names most strongly associated with the Hispanic/Latine community. Learning in a city involved addressing homelessness and "addressing need," while suburbs, towns, and rural areas involved addressing environmental issues and "preserving the character of their small town."
This data elucidates the biases of AI LLM chatbots when discussing learning situations as primarily a white, gender binary, upper middle class pursuit. In addition, when asked to address characteristics of REGS (race, ethnicity, gender, and SES) directly, ChatGPT stitched together stories that illustrated deep structural and societal assumptions and bias. There is more data to show this biased narrative stitching.
Importance
There is both scientific and educational importance to this research. First, this research provides a methodology for interrogating the role of race, ethnicity, gender, and SES in narratives stitched together by AI LLM chatbots through a mixed-methods approach. In doing so, this research illuminates and confirms the whiteness, gender normativity, and class predispositions of AI LLMs when creating stories of learning, providing a window into the biases of AIs, but also our societal and cultural biases as well in learning spaces.
In addition, it calls attention the necessity to engage inservice and preservice teachers in understanding, recognizing, and resisting the underlying assumptions around race, ethnicity, gender, and SES embedded in the AI LLMs and reflective of our society at large, not just about the ethical ramifications of using AI LLMs to cheat or engage in unfair behavior as AI LLMs can serve to reinforce these biases and normative assumptions without direct intervention and facilitating activities by educators.
References
Adler, N. E., Epel, E. S., Castellazzo, G., & Ickovics, J. R. (2000). Relationship of subjective and objective social status with psychological and physiological functioning: Preliminary data in healthy, White women. Health Psychology, 19(6), 586. https://doi.org/10.1037/0278-6133.19.6.586
Cave, S., & Dihal, K. (2020). The Whiteness of AI. Philosophy & Technology, 33(4), 685–703. https://doi.org/10.1007/s13347-020-00415-6
David, ClarissaC., Atun, J., Fille, E., & Monterola, C. (2011). Finding Frames: Comparing Two Methods of Frame Analysis. Communication Methods & Measures, 5(4), 329–351. https://doi.org/10.1080/19312458.2011.624873
Einbeck, J., Darnell, R., & Hinde, J. (2018). npmlreg: Nonparametric Maximum Likelihood Estimation for Random Effect Models (0.46-5) [Computer software]. https://cran.r-project.org/web/packages/npmlreg/index.html
Fields, K. E., & Fields, B. J. (2014). Racecraft: The soul of inequality in American life. Verso.
Foste, Z., & Jones, S. R. (2020). Narrating Whiteness: A Qualitative Exploration of How White College Students Construct and Give Meaning to Their Racial Location. Journal of College Student Development, 61(2), 171–188. https://doi.org/10.1353/csd.2020.0016
Frankenberg, R. (1997). Local Whiteness, Localizing Whiteness. In R. Frankenberg (Ed.), Displacing Whiteness: Essays in Social and Cultural Criticis (pp. 1–34). Duke University Press.
Glaser, B. G. (1965). The Constant Comparative Method of Qualitative Analysis. Social Problems, 12(4), 436–445. https://doi.org/10.2307/798843
Goffman, E. (1974). Frame analysis: An essay on the organization of experience. Harvard University Press.
Jensen, E., Jones, N., Rabe, M., Pratt, B., Medina, L., Orozco, K., & Spell, L. (2021). The Chance That Two People Chosen at Random Are of Different Race or Ethnicity Groups Has Increased Since 2010. Census.Gov. https://www.census.gov/library/stories/2021/08/2020-united-states-population-more-racially-ethnically-diverse-than-2010.html
Marr, B. (2023, May 19). A Short History Of ChatGPT: How We Got To Where We Are Today. Forbes. https://www.forbes.com/sites/bernardmarr/2023/05/19/a-short-history-of-chatgpt-how-we-got-to-where-we-are-today/
Memarian, B., & Doleck, T. (2023). Fairness, Accountability, Transparency, and Ethics (FATE) in Artificial Intelligence (AI) and higher education: A systematic review. Computers and Education: Artificial Intelligence, 5, 100152. https://doi.org/10.1016/j.caeai.2023.100152
Meyer, D., Dimitriadou, E., Hornik, K., Weingessel, A., & Leisch, F. (2023). E1071: Misc Functions of the Department of Statistics, Probability Theory Group (Formerly: E1071), TU Wien (1.7-13) [Computer software]. https://CRAN.R-project.org/package=e1071
NCES. (2006). Status of Education in Rural America. National Center for Education Statistics. https://nces.ed.gov/pubs2007/ruraled/exhibit_a.asp
NCES. (2019). Status and Trends in the Education of Racial and Ethnic Groups. https://nces.ed.gov/programs/raceindicators/index.asp
R Core Team. (2022). R: A Language and Environment for Statistical Computing [Computer software]. R Foundation for Statistical Computing. https://www.R-project.org/
Reddy, M. T. (1998). Invisibility/Hypervisibility: The Paradox of Normative Whiteness. Transformations: The Journal of Inclusive Scholarship and Pedagogy, 9(2), 55–64.
SPARQ. (n.d.). MacArthur Scale of Subjective Social Status – Adult Version. Retrieved August 26, 2023, from https://sparqtools.org/mobility-measure/macarthur-scale-of-subjective-social-status-adult-version/
Viruru, R., & Rios, A. (2021). Needed Methodological Emancipation: Qualitative Coding and the Institutionalization of the Master’s Voice. Qualitative Inquiry, 10778004211021814. https://doi.org/10.1177/10778004211021814
Wyman, C. (2023, June 20). How to Tackle AI—and Cheating—In the Classroom. Wired. https://www.wired.com/story/how-to-tackle-ai-and-cheating-in-schools-classroom/
Yang, J. (2023, January 14). Educators worry about students using artificial intelligence to cheat. PBS NewsHour. https://www.pbs.org/newshour/show/educators-worry-about-students-using-artificial-intelligence-to-cheat
Session specifications
Digital Citizen Advocate
- Support educators and students to critically examine the sources of online media and identify underlying assumptions.
Equity and Citizenship Advocate
- Ensure all students have skilled teachers who actively use technology to meet student learning needs.
Citizen
- Establish a learning culture that promotes curiosity and critical examination of online resources and fosters digital literacy and media fluency.