Teacher-Driven, Algorithm-Supported: Getting More Out of Adaptive Learning Tools
,
Colorado Convention Center, 108/10/12
Research papers are a pairing of two 18 minute presentations followed by 18 minutes of Discussion led by a Discussant, with remaining time for Q & A.
This is presentation 2 of 2, scroll down to see more details.
Other presentations in this group:
Presenters
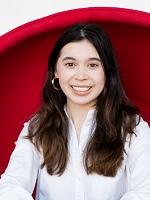
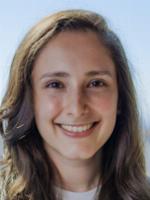
Session description
Framework
Our investigation of stakeholder perspectives on the value of ALTs relied on a definition of value from the Teacher Response Model (TRM), a research-based model of teachers’ decision-making process for technology implementation in the classroom (Kopcha et al., 2020). In contrast with the existing implementation frameworks and research, which consider value only in terms of students’ constructivist, authentic learning, and achievement outcomes, this model conceptualizes the value of education technologies more broadly. Namely, TRM posits that teachers may also find value in technologies because they support a wide variety of their needs as educators, such as efficiency and effectiveness in their administrative tasks, classroom learning management, and communication with families. Kopcha and colleagues propose that a key component of teachers’ perceptions of technology’s value in the classroom depends on how well it helps them complete their various responsibilities, including routine work tasks and tasks that support students’ learning needs. Using conceptions of value in the TRM, our research question explores what value (and challenges) teachers, teacher support staff, and EdTech developers perceive ALTs provide for both students and teachers.
Methods
The TRM’s expansive definition of value encouraged us to take an interpretive qualitative approach to the research design to understand how those involved with a phenomena (ALTs) interpret and construct meaning around their experiences (Merriam & Tisdell, 2015). For our research design, this meant collecting a wider range of stakeholder perspectives regarding the value of ALTs compared to prior studies, without any specific hypotheses about which aspects of value would be salient for individuals in the three stakeholder groups. This also meant we would need to collect data in a systematized and open-ended way. For this reason, the research team utilized a semi-structured interview method for data collection to elicit participant perspectives in their own words. Thematic analysis was used to categorize and interpret varying perspectives to provide a rich narrative around the use and value of ALTs in K-12 education. The research design, study materials, and procedures were reviewed and approved by an Institutional Review Board.
Participants were recruited through our network of education professionals using media posts, snowball sampling, and cold calls via contact pages of EdTech company websites, professional websites (e.g., LinkedIn), and industry conferences (e.g, ISTE). We focused recruitment on five professions that would allow us to capture a holistic set of perspectives surrounding the development, integration, and use of ALTs (i.e., teachers, teacher educators, school administrators, technology coaches, EdTech developers). Our final sample included 25 participants, with 5 Teachers (20%), 7 Teacher Support professionals (28%), and 13 EdTech professionals (52%).
Interviews lasted one hour and were conducted over Zoom. Participants’ audio and/or video were recorded over Zoom in accordance with the participant’s preference, as outlined on their consent forms. Participants were sent a $25 gift card as compensation.
Results
Analysis resulted in three overarching concepts (i.e., learning management, student agency and engagement, and implementation challenges), under which themes regarding stakeholder perspectives on the advantages and challenges of ALTs could be organized and contrasted with one another. Learning management themes suggest that stakeholders perceive features such as real-time student data and tailored learning content as creating value for teachers by supporting efficiency in their learning management, however that value is impacted by stakeholders’ concerns with ALT grading and data collection processes. Student agency and engagement themes highlight how certain user interaction features can create value or challenges for learners depending on whether the features were designed with students’ developmental, and competence needs in mind. Finally, the implementation challenges themes suggest that for ALTs to create value in K-12 settings, stakeholders need better alignment around their ALT implementation goals and expectations. We leverage these data to make recommendations for future research and development so stakeholders can maximize the affordances of ALTs for K-12 students and teachers.
Importance
Education research journals have seen an increase in the number of articles focused on the design, implementation, and evaluation of ALTs for effective learning. However, much of this research has focused on students in higher education with a small amount of research examining K-12 settings relative to the popularity of ALTs in those settings. Even more rare are comprehensive studies of the advantages and challenges of these technologies from the perspective of the education stakeholders involved in both the design and implementation of these tools.
This study aimed to address this gap by conducting interviews with Teachers, Teacher Support staff, and Education Technology professionals to develop a comprehensive understanding of their perceptions of the value they associate with the use of ALTs in K-12 education. We present our findings in conversation with current ALT literature and offer recommendations for how practitioners in education and EdTech fields can further improve and integrate these tools in K-12 classrooms.
References
Alam, A. (2021). Should Robots Replace Teachers? Mobilisation of AI and Learning Analytics in Education. 1–12. https://doi.org/10.1109/ICAC353642.2021.9697300.
Asaad, D. (2017). Personalized Adaptive Learning Impact on the Student Experience: Stakeholders’ Perspectives. National American University.
Bingham, A. J., Pane, J. F., Steiner, E. D., & Hamilton, L. S. (2018). Ahead of the Curve: Implementation Challenges in Personalized Learning School Models. Educational Policy, 32(3), 454–489. https://doi.org/10.1177/0895904816637688
Boddle Learning. (n.d.). Boddle [Computer software]. https://www.boddlelearning.com/
Brasca, C., Krishnan, C., Marya, V., Owen, K., Sirois, J., & Ziade. (2022). Technology is shaping learning in higher education. McKinsey. https://www.mckinsey.com/industries/education/our-insights/how-technology-is-shaping-learning-in-higher-education
Braun, V., & Clarke, V. (2006). Using thematic analysis in psychology. Qualitative Research in Psychology, 3(2), 77–101. https://doi.org/10.1191/1478088706qp063oa
Brinkmann, S. (2015). Perils and Potentials in Qualitative Psychology. Integrative Psychological and Behavioral Science, 49(2), 162–173. https://doi.org/10.1007/s12124-014-9293-z
Cavanagh, T., Chen, B., Lahcen, R. A. M., & Paradiso, J. (2020). Constructing a Design Framework and Pedagogical Approach for Adaptive Learning in Higher Education: A Practitioner’s Perspective. The International Review of Research in Open and Distributed Learning, 21(1), 172–196. https://doi.org/10.19173/irrodl.v21i1.4557
Conger, A. J. (1980). Integration and Generalization of Kappas for Multiple Raters. Psychological Bulletin, 88(2), 322–328. https://doi.org/10.1037/0033-2909.88.2.322
Conjuguemos. (n.d.). Conjuguemos [Computer software]. https://conjuguemos.com/
Curriculum Associates. (n.d.). IReady Learning [Computer software]. https://www.curriculumassociates.com/
De Santana, S. J., Paiva, R., Bittencourt, I. I., Ospina, P. E., De Amorim Silva, R., & Isotani, S. (2016). Evaluating the impact of mars and venus effect on the use of an adaptive learning technology for Portuguese and mathematics. Proceedings - IEEE 16th International Conference on Advanced Learning Technologies, ICALT 2016, 31–35. https://doi.org/10.1109/ICALT.2016.58
Dedoose (4.12). (2020). [Computer software]. SocioCultural Research Consultants, LLC. https://www.dedoose.com/
DreamBox Learning. (n.d.). DreamBox Math [Computer software]. https://www.dreambox.com/solutions/math
Duolingo. (n.d.). Duolingo [Computer software]. https://www.duolingo.com/
Edmentum. (n.d.). Edmentum [Computer software]. https://www.edmentum.com/products
EdSurge. (2016). Decoding Adaptive (pp. 1–33). Pearson.
Ekowo, M., & Palmer, I. (2016). The Promise and Peril of Predictive Analytics in Higher Education: A Landscape Analysis. New America.
El-Sabagh, H. A. (2021). Adaptive e-learning environment based on learning styles and its impact on development students’ engagement. International Journal of Educational Technology in Higher Education, 18(1). https://doi.org/10.1186/S41239-021-00289-4
Forsyth, B., Kimble, C., Birch, J., Deel, G., & Brauer, T. (2016). Maximizing the adaptive learning technology experience. Journal of Higher Education Theory and Practice, 16(4), 80–88.
Gergen, K. J., Josselson, R., & Freeman, M. (2015). The promises of qualitative inquiry. American Psychologist, 70(1), 1–9. https://doi.org/10.1037/a0038597
Harati, H., Sujo-Montes, L., Tu, C.-H., Armfield, S. J. W., & Yen, C.-J. (2021). Assessment and Learning in Knowledge Spaces (ALEKS) Adaptive System Impact on Students’ Perception and Self-Regulated Learning Skills. Education Sciences, 11(10), Article 10. https://doi.org/10.3390/educsci11100603
Houghton Mifflin Harcourt. (n.d.-a). Read 180 [Computer software]. https://www.hmhco.com/programs/read-180
Houghton Mifflin Harcourt. (n.d.-b). System 44 [Computer software]. https://www.hmhco.com/programs/system-44#overview
Hughes, J. E. (2016). Learning Across Boundaries: Educator and Startup Involvement in the Educational Technology Innovation Ecosystem. Contemporary Issues in Technology and Teacher Education, 19(1), 62–96.
Imagine Learning. (n.d.-a). Imagine Espanol [Computer software]. https://www.imaginelearning.com/products/spanish-language-arts/espanol/
Imagine Learning. (n.d.-b). Imagine Language & Literacy [Computer software]. https://www.imaginelearning.com/products/ela/language-and-literacy/
Imagine Learning. (n.d.-c). Imagine Math [Computer software]. https://www.imaginelearning.com/products/math/math/
James, L. A. (2012). Evaluation of an Adaptive Learning Technology as a Predictor of Student Performance in Undergraduate Biology. Appalachian State University.
Johnson, B. G., Phillips, F., & Chase, L. G. (2008). An Intelligent Tutoring System for the Accounting Cycle: Enhancing Textbook Homework with Artificial Intelligence. SSRN. https://doi.org/10.2139/SSRN.1151791
Kelly, D. (2008). Adaptive versus Learner Control in a Multiple Intelligence Learning Environment. Journal of Educational Multimedia and Hypermedia, 17(3), 307-336. Waynesville, NC USA: Association for the Advancement of Computing in Education (AACE). https://www.learntechlib.org/primary/p/24252/.
Kerr, P. (2016). Adaptive learning. ELT Journal, 70(1), 88–93. https://doi.org/10.1093/elt/ccv055
Keuning, T., & Van Geel, M. (2021). Differentiated Teaching with Adaptive Learning Systems and Teacher Dashboards: The Teacher Still Matters Most. IEEE Transactions on Learning Technologies, 14(2), 201–210. https://doi.org/10.1109/TLT.2021.3072143
Khan Academy. (n.d.). Khan Academy [Computer software]. https://www.khanacademy.org/
Knowre. (n.d.). Knowre Math [Computer software]. https://www.knowre.com/
Koedinger, K. R., & Aleven, V. (2007). Exploring the Assistance Dilemma in Experiments with Cognitive Tutors. Educational Psychology Review, 19, 239–264. https://doi.org/10.1007/s10648-007-9049-0
Kolchenko, V. (2018). Can Modern AI replace teachers? Not so fast! Artificial Intelligence and Adaptive Learning: Personalized Education in the AI age. HAPS Educator, 22(3), 249–252. https://doi.org/10.21692/haps.2018.032
Kooapps. (n.d.). Pictoword [Computer software]. https://pictoword.app/
Kopcha, T. J., Neumann, K. L., Ottenbreit-Leftwich, A., & Pitman, E. (2020). Process over product: The next evolution of our quest for technology integration. Educational Technology Research and Development, 68(2), 729–749. https://doi.org/10.1007/s11423-020-09735-y
Kurtz, H., Lloyd Sterling, Harwin Alex, Chen Xinchun, & Furuya Yukiko. (2019). Personalized Learning: Results of a National Survey. Editorial Projects in Education. www.edweek.org/rc
Landis, J. R., & Koch, G. G. (1977). The Measurement of Observer Agreement for Categorical Data. Biometrics, 33(1), 159–174. https://doi.org/10.2307/2529310
Learn Without Limits. (n.d.). Moby Max [Computer software]. https://www.mobymax.com/
Lexia Learning. (n.d.). Lexia [Computer software]. https://www.lexialearning.com/
Linacre, J. M. (2000). Computer-Adaptive Testing: A Methodology Whose Time Has Come. In S. Chae, U. Kang, E. Jeon, & J. M. Linacre (Eds.), Development of computerized middle school achievement tests. Komesa Press.
Liu, M., Kang, J., Zou, W., Lee, H., Pan, Z., & Corliss, S. (2017). Using Data to Understand How to Better Design Adaptive Learning. Technology, Knowledge and Learning, 22(3), 271–298. https://doi.org/10.1007/s10758-017-9326-z
Lowendahl, J.-M., Morgan, G., & Thayer, T.-L. (n.d.). Top 10 Strategic Technologies Impacting Higher Education in 2016. Gartner. Retrieved August 14, 2023, from https://www.gartner.com/en/documents/3186323
Martin, F., Chen, Y., Moore, R. L., & Westine, C. D. (2020). Systematic review of adaptive learning research designs, context, strategies, and technologies from 2009 to 2018. Educational Technology Research and Development, 68(4), 1903–1929. https://doi.org/10.1007/s11423-020-09793-2
McDonald, N., Schoenebeck, S., & Forte, A. (2019). Reliability and Inter-rater Reliability in Qualitative Research: Norms and Guidelines for CSCW and HCI Practice. Proceedings of the ACM on Human-Computer Interaction, 3(CSCW), 72:1-72:23. https://doi.org/10.1145/3359174
McGraw Hill. (n.d.). ALEKS [Computer software]. https://www.aleks.com/
McIntosh, M. J., & Morse, J. M. (2015). Situating and Constructing Diversity in Semi-Structured Interviews. Global Qualitative Nursing Research, 2. https://doi.org/10.1177/2333393615597674
Merriam, S. B., & Tisdell, E. J. (2015). Qualitative Research: A Guide to Design and Implementation. John Wiley & Sons.
Milman, N. B., & Henderson, J. (2021). Preliminary COVID-19 Pandemic Lessons Learned from Educational Technology Coaches, Coordinators, and Specialists. International Society for Technology in Education Conference.
MIND Research Institute. (n.d.). ST Math [Computer software]. https://www.stmath.com/
Mojarad, S., Essa, A., Mojarad, S., & Baker, R. S. (2018). Studying Adaptive Learning Efficacy using Propensity Score Matching. Proceedings of the 8th International Conference on Learning Analytics and Knowledge (LAK18), December, 1–8.
Molenaar, I., & Knoop-Van Campen, C. A. N. (2019). How Teachers Make Dashboard Information Actionable. IEEE Transactions on Learning Technologies, 12(3), 347–355. https://doi.org/10.1109/TLT.2018.2851585
Moltudal, S., Høydal, K., & Krumsvik, R. J. (2020). Glimpses Into Real-Life Introduction of Adaptive Learning Technology: A Mixed Methods Research Approach to Personalised Pupil Learning. Designs for Learning, 12(1), 13–28. https://doi.org/10.16993/dfl.138
Panorama Education. (n.d.). Panorama [Computer software]. https://www.panoramaed.com/
Pantelimon, F.-V., Bologa, R., Toma, A., & Posedaru, B.-S. (2021). The Evolution of AI-Driven Educational Systems during the COVID-19 Pandemic. Sustainability, 13(23), Article 23. https://doi.org/10.3390/su132313501
Renaissance. (n.d.). Freckle Math [Computer software]. https://freckle.com/en-us/
Roberts-Mahoney, H., Means, A. J., & Garrison, M. J. (2016). Netflixing human capital development: Personalized learning technology and the corporatization of K-12 education. Journal of Education Policy, 31(4), 405–420. https://doi.org/10.1080/02680939.2015.1132774
Saga Education. (n.d.). Woot Math [Computer software]. https://www.wootmath.com/
Savvas Learning Company. (n.d.). SuccessMaker [Computer software]. https://www.savvas.com/index.cfm?locator=PS2qJ3
Smith, K. (2018). Perceptions of preservice teachers about adaptive learning programs in K-8 mathematics education. Contemporary Educational Technology, 9(2), 111–130. https://doi.org/10.30935/cet.414780
Twining, P., Heller, R. S., Nussbaum, M., & Tsai, C.-C. (2017). Some guidance on conducting and reporting qualitative studies. Computers & Education, 106, A1–A9. https://doi.org/10.1016/j.compedu.2016.12.002
Valsiner, J. (2014). Needed for cultural psychology: Methodology in a new key. Culture & Psychology, 20(1), 3–30. https://doi.org/10.1177/1354067X13515941
Walkington, C. A. (2013). Using adaptive learning technologies to personalize instruction to student interests: The impact of relevant contexts on performance and learning outcomes. Journal of Educational Psychology, 105(4), 932–945. https://doi.org/10.1037/a0031882
Walkington, C. A., & Sherman, M. (2012). Using Adaptive Learning Technologies to Personalize Instruction: The Impact of Interest-Based Scenarios on Performance in Algebra. The Future of Learning: Proceedings of the 10th International Conference of the Learning Sciences (ICLS 2012) – Volume 1, Full Papers, 80–97.
Whizz Education. (n.d.). Maths-Whizz [Computer software]. https://whizz.com/en-us/
Yilmaz, B. (2017). Effects of Adaptive Learning Technologies on Math Achievement: A Quantitative Study on ALEKS Math Software. University of Missouri-Kansas City.
Zearn. (n.d.). Zearn Math [Computer software]. https://www.zearn.org/
Session specifications
Learning Designer
- Help educators use digital tools to create effective assessments that provide timely feedback and support personalized learning.
Leader
- Shape, advance and accelerate a shared vision for empowered learning with technology by engaging with education stakeholders.
- Use technology to design and implement a variety of formative and summative assessments that accommodate learner needs, provide timely feedback to students and inform instruction.